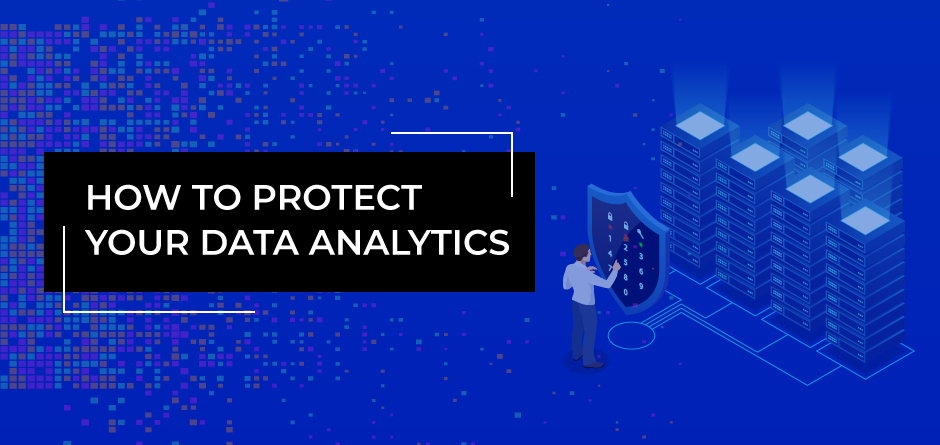
How To Protect Your Data Analytics
The data analysis is so robust that you can combine data sets to infer the lifestyle, consumer habits, social media preferences of the user even if no data set reveals this information. It’s no wonder that people have raised concerns about the impact of big data on privacy. People worry that essential protection is now being challenged by the sheer speed, accuracy, and amount of data and how it can be manipulated once taken for granted. Some people believe that our concept of privacy must be changed and that the need for innovation and unlocking the value of data must exceed traditional concepts. But this idea of a compromise between privacy and innovation is useless and outdated. While businesses are using data analysis to reveal new insights and innovations to drive progress, it is entirely possible to protect personal privacy. Just as technology has produced data analysis, it can also be used to solve the resulting privacy issues. Data analysis aims to coordinate the needs of solid data protection and data-driven innovation technology because it requires a lot of processing power, so the solution must be lightweight.
In recent years, the rapid technological advancement of intelligent devices and their use in a wide range of applications has caused the data generated by these devices to grow exponentially. Therefore, traditional data analysis techniques may not handle this extreme data called big data generated by different devices. However, this exponential data growth has opened the door for different types of attackers to launch various attacks exploiting various vulnerabilities in the data analysis process. Taking inspiration from the previous discussion, we explore models and techniques based on machine learning and deep learning that can identify and mitigate known and unknown attacks in this article. Machine learning and deep learning-based technology can use test and training data sets across many network domains to learn from traffic patterns and make intelligent decisions about attack identification and mitigation. Here are a few examples mentioned below:
1. Image Recognition
Image recognition may be a well-known and widespread example of machine learning within the world. It can identify an object as a digital image, supporting the intensity of the pixels in black and white images or color images.
Real-world examples of image recognition:
- Label an x-ray as cancerous or not.
- Recognize handwriting by segmenting one letter into smaller images.
- Machine learning is also frequently used for facial recognition within an image. Using a database of individuals, the system can identify commonalities and match them to faces. This is often used in law enforcement.
2. Speech recognition
Machine learning can translate speech into text. Specific software applications can convert live voice and recorded speech into a document. The speech is often segmented by intensities on time-frequency bands also.
Real-world examples of speech recognition:
- Voice search
- Voice dialing
- Appliance control
Some of the foremost common uses of speech recognition software are devices like Google Home or Amazon Alexa.
3. Medical diagnosis
Machine learning can assist health professionals with the diagnosis of diseases. Many professionals use a combination of chatbots and speech recognition features to detect patterns in symptoms.
Real-world examples for medical diagnosis are:
- We are assisting in formulating a diagnosis or recommending a treatment option.
- Oncology and pathology make use of ML to identify cancerous tissue.
- Analyze bodily fluids.
- As far as rare diseases are concerned, a combination of face recognition software and ML helps scan pictures of a patient and detect phenotypes that correlate with rare genetic disorders.
A secure data analysis architecture based on deep learning and machine learning has been proposed to classify normal or attacked input data. The detailed classification of the security data analysis is summarized as a threat model. The threat model uses multiple parameters such as efficiency, delay, accuracy, reliability, and attacks to solve various research challenges in analyzing security data. Finally, a comparison of existing safety data analysis recommendations is entered for multiple parameters, allowing the end-user to select one of the safety data analysis recommendations and compare it with other suggestions. Data is a term used to refer to massive data sets with more diverse and complex structures. These characteristics are often associated with additional difficulties in storing, analyzing, and applying other procedures or extracting results. Data analysis is a term used to describe the process of studying large amounts of complex data to reveal hidden patterns or identify secret associations. However, there are apparent contradictions between the security and privacy of big data and its widespread use. This document concentrates on privacy and security issues in big data, the difference between the two, and privacy requirements in big data. Many privacy protection mechanisms have been developed for privacy protection at different stages of the big data life cycle. This document aims to provide an essential review of the privacy protection mechanisms in big data and to challenge existing tools. This article also introduces recent privacy protection technologies in big data, such as finding a needle in a haystack, identity-based anonymization, differential privacy, privacy-protected big data release, and rapid anonymization of big data streams. This article is about the privacy and security aspects of healthcare in big data. It also conducts comparative research on several newer big data privacy technologies.
In today’s digital world, a large amount of information is stored in big data, and database analysis can provide opportunities to solve important social problems, such as healthcare. Smart energy big data analytics is also a very complex and challenging topic, having many common problems with big data analytics in general. Smart energy big data involves a wide range of physical processes, among which data intelligence can have a major impact on the safe operation of the system in real-time. This is also helpful for marketing companies and other business ventures to develop their business. Because the database contains personal information, it is easy to provide direct access to researchers and analysts.
Data analytics has appealed to many organizations because they lack standard security and privacy protection tools, and a large portion of them choose not to use these services. These sections discuss possible strategies for updating the big data platform with the help of privacy protection features. Developers must be able to verify that their applications comply with privacy agreements and regardless of changes in applications or privacy regulations, sensitive information is kept confidential. To overcome these challenges, it was concluded that more contributions in formal methods and test procedures are required.
One platform that securely processes your data is Microsoft Power BI. It keeps your data secure with industry-leading data protection features like sensitivity labeling, real-time access monitoring, end-to-end encryption, and more. If you’re looking to implement a BI platform for your business, get in touch with us.
RECENT POSTS
CATEGORIES